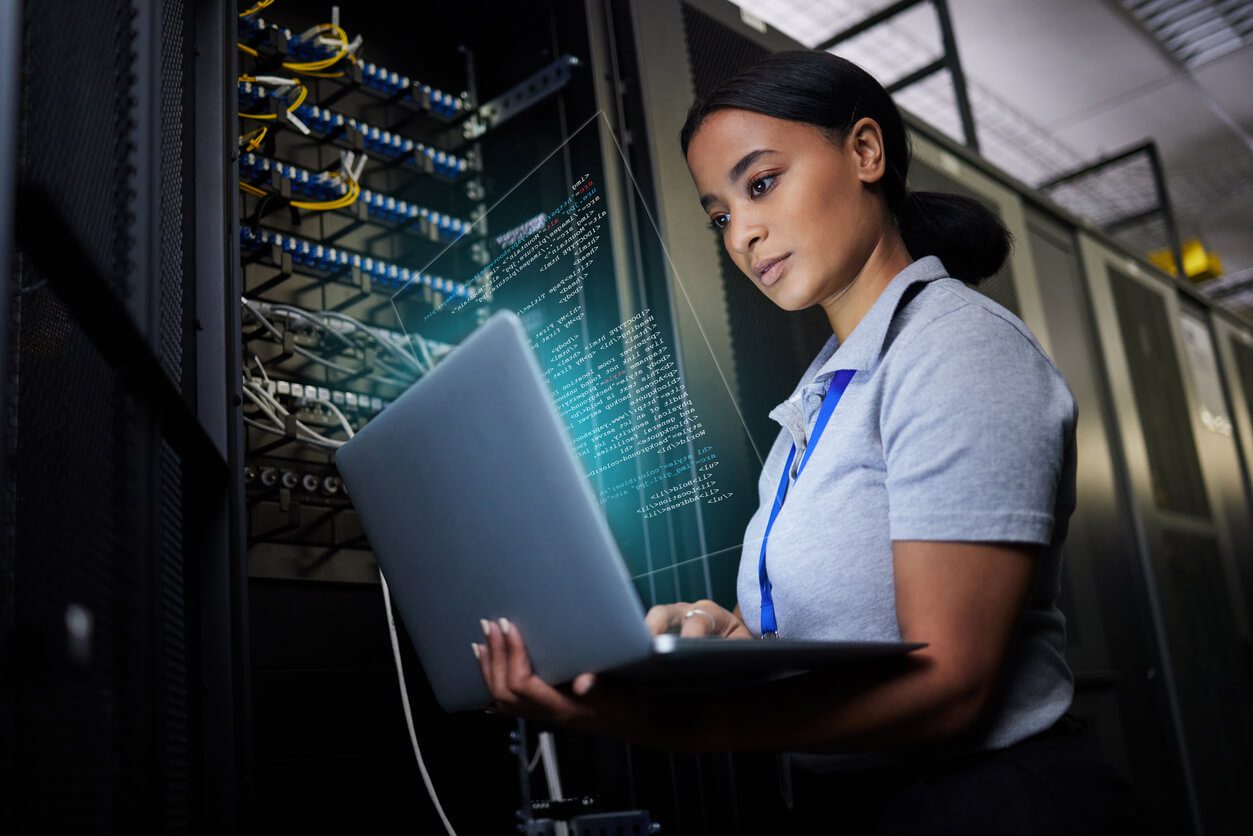
How AI and Machine Learning are Evolving DevOps
The introduction of AI and machine learning has changed the way we perceive DevOps. Many Software companies have made use of AI and ML with DevOps to ensure the proper functioning and delivery of high-quality products to their clients. The most common prediction is that AI and ML will be used in huge volumes in all the areas of DevOps. AI and ML are perfect fits for a DevOps culture. They can process vast amounts of information and help perform menial tasks, freeing the IT staff to do more targeted work. They can learn patterns, anticipate problems and suggest solutions. If DevOps’ goal is to unify development and operations, AI and ML can smooth out some of the tensions that have divided the two disciplines in the past.
“AI is one of the most important things humanity is working on,” Google CEO Sundar Pichai said earlier this year. “It is more profound than, I dunno, electricity or fire.”
In simple words, AI is an extensive concept of computers being able to complete a task to reduce the human burden. On the other hand, ML is an application of AI, which allows machines to learn from large data. Effective web search, voice recognition, automated cars, games are some of the fields that have applied ML to improve user experience.
Now let’s understand what is DevOps.
DevOps is a set of practices that includes Development (Dev) and Operations (Ops), it is the union of process, people, and technology to ensure continuous delivery of values to customers. For example, a company has a wide range of products that are being used by customers from all over the world. To keep up with the customer’s requirements and complaints, the development and operations team must work together. DevOps thus ensures fast and short release cycles that can get new applications and features to customers quickly and reliably. It is also about team coordination, feedback loops for continuous improvement, this helps in capturing the release bugs as early as possible thus reducing the testing time.
When DevOps combines with AI, there are many functions that fall off the shoulders of the human. Here are six ways, according to Mike Hughes, AI and ML can and will change DevOps for the better.
Promoting Feedback on Performance
One of the key tenets of DevOps is the use of continuous feedback loops at every stage of the process. This includes using monitoring tools to provide feedback on the operational performance of running applications. This is one area today where ML is impacting DevOps already. Monitoring platforms gather massive amounts of data in the form of performance metrics, log files, and other types. Advanced monitoring platforms are applying machine learning to these datasets to proactively identify problems very early and make recommendations. These recommendations go to the DevOps team members so that they can ensure that the application service remains viable. Machine learning is enhancing the continuous feedback loops that are critical to DevOps.
Enabling Communication
Communication and feedback are always one of the biggest challenges when organizations move to a DevOps methodology. Human interaction is vital, but with so much information flowing through the system, teams need to set up a wider variety of channels to set and revise workflows on the fly. Using automation technology, chatbots, and other systems initiated by AI, these communications channels can become more streamlined and more proactive.
Correlate Data Across Platforms and Tools
To operate efficiently, DevOps teams need to simplify tasks. This is getting more difficult as environments get more complex. Start with monitoring tools. Teams tend to use multiple tools that monitor an application’s health and performance in different ways. Machine learning applications can absorb these data streams and find correlations, giving the team a more holistic view of the application’s overall health.
Manage a Flurry of Alerts
Since DevOps encourages teams to “fail but fail fast,” it’s critical to have an alert system that spots a flaw quickly. This tends to create scenarios where alerts are coming fast and furious, all labeled with the same severity, making it difficult for teams to react. Machine learning applications can help teams prioritize their responses based on factors such as past behavior, the magnitude of the current alert, and the source that specific alerts are coming from. Humans can set up rules, but machines can help manage these types of situations when too much data overwhelms the system.
Evaluating Past Performance
AI/ML also has the potential to help developers during the application creation process. By examining the success of past applications in terms of build/compile success, successful testing completion, and operational performance, machine learning algorithms could make recommendations to developers proactively based on the code they are writing or the application that they are building. The AI engine could direct the developer on how to build the most efficient and highest-quality application.
Software Testing
In the future, we could see AI/ML applied to other stages of the software development life cycle to provide enhancements to a DevOps methodology or approach. One area where this may happen could be in the area of software testing. Unit tests, regression tests, functional tests, and user acceptance tests all produce large amounts of data in the form of test results. Applying AI or machine learning algorithms to these test results could identify patterns of poor coding practices that result in too many errors caught by the tests. This information could then inform the development teams so that they can become more efficient in the future.
Similarly, leveraging historical data, AI/ML could be used to fine-tune deployment strategies as applications are moved from Dev to Test to Production environments.
Not only are these but they other aspects where AI and ML can be applied to DevOps culture like:
Optimizing functions of a DevOps environment
DevOps and Machine Learning share a strong alliance with related capabilities like predictive analysis, algorithmic IT operations, Operations Analytics, and AI. The introduction of Machine Learning into DevOps has brought benefits like checking highly complex data sets. Detect patterns and anti patterns, uncover new ideas, repeat and refine queries with speed and perfection. For instance, delivery processes are often tracked with various DevOps tools, these tools produce tons of knowledge, and any quiet error during this data is often detected by the appliance of Machine Learning. Large code volume, slow-release rate, and long built times are a number of the problems which will be put in restraint using Machine Learning. Machine Learning also can review the QA results and find out errors and present a far better testing pattern that supported these findings. It can track the pattern within the behavior of the event and operations user and detect irregularities indicating malicious activities like stealing intellectual properties, deploying authorized code, etc.
Management of codes can become easy and efficient with the assistance of Machine Learning. Large data volumes, transaction records, and therefore the number of users is often analyzed by Machine Learning. It can mean errors concerning normal values. It also can detect errors within the normal proceedings, and wisely analyze previously released logs to seek out the problems with the new release. ML can read the previously used good patterns and plan out the simplest configuration to maximize performance.
Tracking user behavior and security
AI and ML can help us optimize our application by analyzing usage data and security threats. It can identify the modules and functionalities of an application that are getting used the foremost so that we will focus our efforts on improving user experience in those areas. With the specialization in user behavior, AI can help us to prioritize the user experience in our release planning. Tracking user behavior and making user experience-related decisions is the most vital part of a product’s success. By leveraging the method, AI and ML eliminates the human efforts within the process and helps organizations to form it more efficiently and accurately. With the assistance of AI, we can easily track the safety threats and fortify our defenses to combat the attack before it puts the organization into any loss.
Accelerates automation
AI automation and consistency into the DevOps and thus within the release process, but some areas require human efforts to manage the method. AI helps us to automate the processes which will reduce the probabilities of human errors. This automation process also helps us to release valuable resources to be utilized in innovative solutions. AI has the potential of self-heal problems, it can recommend solutions for writing more efficient and performing codes. It helps the event team to decide on what to be addressed next by prioritizing the anticipated impact of a change.
There are many websites that help you do AI and ML with DevOps. Using one of them could help your business change for the better. One such website is Lorhan Corp.
They are capable of driving Frictionless Application Development and Automated Operations with DevOps. Lorhan helps customers do DevOps maturity assessment and propose appropriate industry-standard tools. These tools help agile DevOps teams to govern, test, and monitor software while achieving an amazing user experience. This Solution provides any business IT organization a platform to deliver IT transformation that has been promised to business. This is a collaborative approach that engages people and enables them to establish interaction between processes and the integration of tools. The platform enables the organization to deliver on the promise of agile by easing any limitations that might exist due to practices that have been followed by the operation organization.